The Security Risks of AI Models: Hacking Risks and Mitigation
- Samuel Ventimiglia
- Feb 22, 2024
- 2 min read
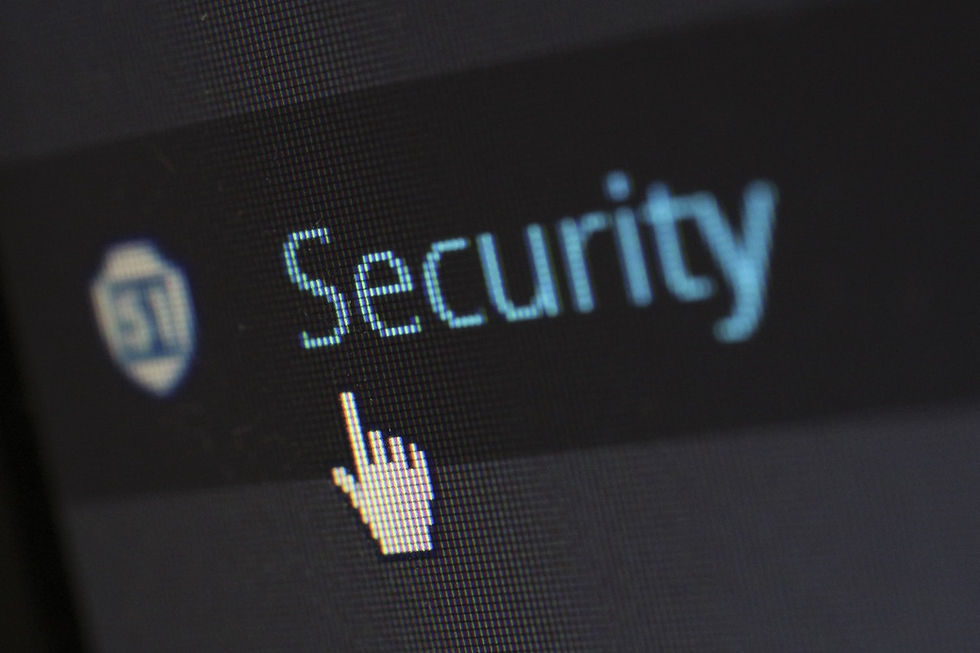
Hacking Risks and Mitigation of an AI model is one of the most important thing to keep in mind if you want to operate in this world.
Artificial Intelligence (AI) has undoubtedly revolutionised various industries, offering unprecedented advancements in automation, efficiency, and problem-solving. However, with great power comes great responsibility, and the deployment of AI models also brings forth an array of security risks that demand careful consideration. In this blog post, we will explore some of the significant security concerns associated with AI models and strategies to mitigate these risks.
1. Adversarial Attacks:
One of the primary security challenges in the realm of AI models is adversarial attacks. These attacks involve manipulating the input data to deceive the model into making incorrect predictions. By introducing subtle modifications that are imperceptible to the human eye, attackers can cause AI systems to misclassify images, misinterpret language, or generate flawed outcomes.
Mitigation Strategy: Implementing robust input validation, using adversarial training techniques, and regularly updating models with new data can help enhance resistance against adversarial attacks.
2. Data Privacy and Bias:
AI models heavily rely on vast datasets for training, and these datasets often contain sensitive or personal information. Ensuring data privacy is crucial to prevent unauthorised access and potential misuse. Additionally, bias in training data can lead to biased model predictions, which may have ethical implications and exacerbate existing social disparities.
Mitigation Strategy: Employing privacy-preserving techniques such as federated learning, and differential privacy, and conducting thorough audits of training data to identify and rectify biases can help address data privacy and bias concerns.
3. Model Inversion and Extraction:
Model inversion involves reconstructing sensitive information from an AI model's outputs, and model extraction involves replicating the model itself. Both pose serious threats to intellectual property and user privacy, as attackers could gain access to proprietary algorithms or sensitive training data.
Mitigation Strategy: Employing secure model architectures, implementing access controls, and encrypting both the model parameters and the training data can help safeguard against model inversion and extraction.
4. Lack of Explainability:
Many AI models, especially deep neural networks, are often considered as "black boxes" due to their complex structures. This lack of transparency raises concerns about the interpretability of model decisions, making it challenging to understand how and why a specific prediction was made.
Mitigation Strategy: Utilising explainable AI techniques, such as LIME (Local Interpretable Model-agnostic Explanations) or SHAP (SHapley Additive exPlanations), can enhance model interpretability and foster trust in AI systems.
As AI continues to advance, addressing the security risks associated with these models becomes paramount. By adopting a proactive and multi-faceted approach that combines robust cybersecurity practices, privacy preservation, and ethical considerations, we can harness the power of AI while minimizing the potential risks. As stewards of this transformative technology, it is our responsibility to ensure that AI is developed and deployed ethically, with the security and well-being of users at the forefront.
Comentários